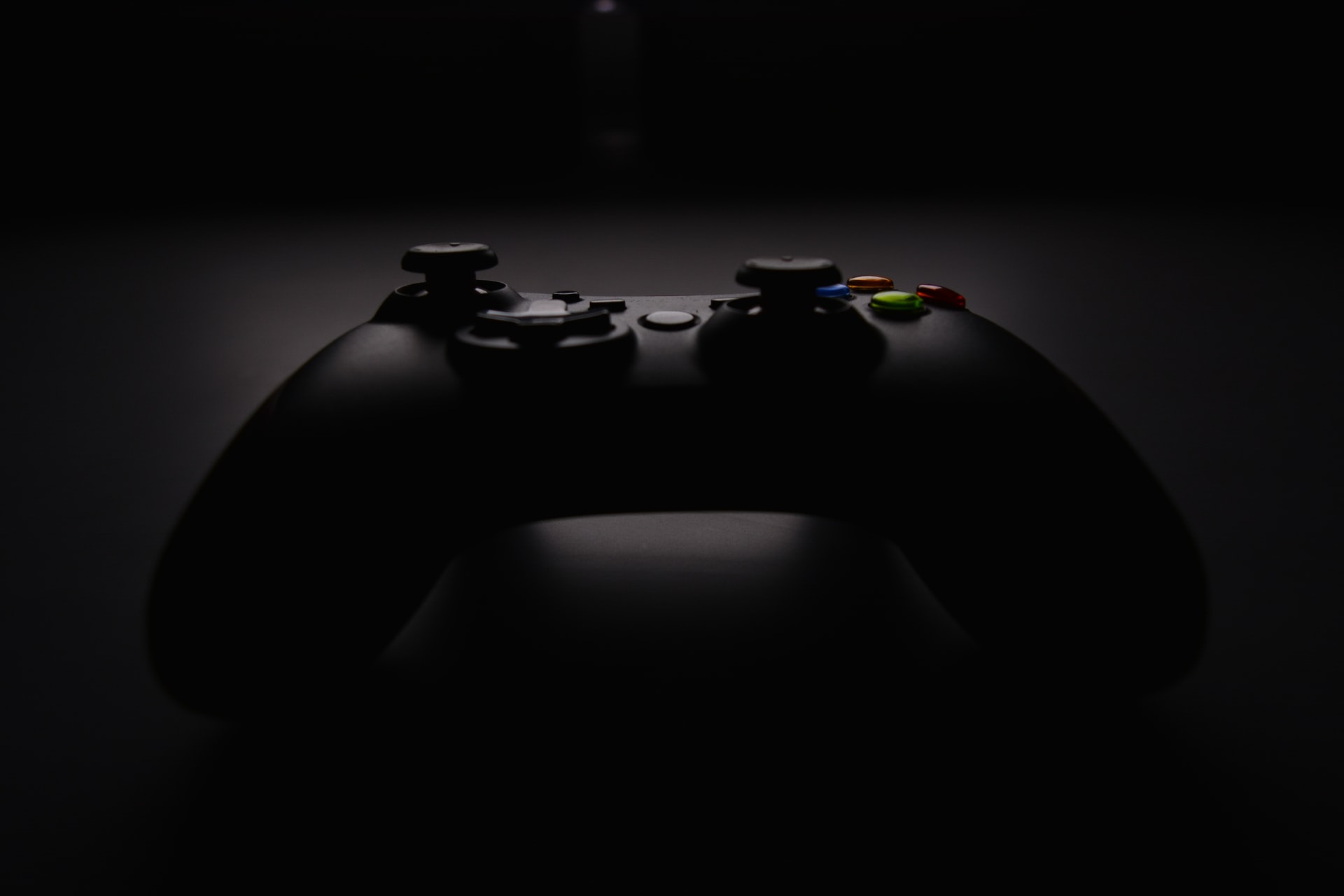
Artificial Intelligence (AI) companies working for a long time have developed a Reinforced Learning (RL) method in games. Thanks to this system, which makes the games much more enjoyable, weak players will perform well.
Thanks to a system developed by DeepMind, the Artificial Intelligence (AI) company within Google’s parent company Alphabet, much stronger reactions can be given in games. In today’s world, where Artificial Intelligence (AI) is involved in almost every aspect of our lives, games cannot be considered independent of real life. Being aware of this, Google company will use Artificial Intelligence (AI) to increase the skill level in competitions.
Where Can The Reinforced Learning (RL) Method Be Used in Games?
This Artificial Intelligence (AI) system developed by Google can be used in many areas. Especially games such as chess provide much more convenience in such matters.
With a simple example, let’s say that there are 14 decision points to the 10th power in Heads–Up Limit Texas Hold’em, and this number increases to 10 to the 170th in Go. To escape all this, a method called Reinforced Learning (RL) was preferred.
The structure proposed by the company officials is known as the Monte Carlo Tree Survey (ABR IS–MCTS). Capable of being translated towards the best response based on knowledge/situation. The actors here follow an algorithm to play a game. ABR IS–MCTS makes several attempts to learn how to create an accurate and exploitable counter–strategy intuitively.
This Artificial Intelligence (AI), which can learn with envelopes, allows players to be at a level to make the best moves. Thus, the games can become much more enjoyable. Further development of this method is expected in the coming years.
Reinforced Learning (RL) remains the new gold standard for creating intelligent video games Artificial Intelligence (AI). The main advantage of Reinforced Learning (RL) over traditional competition Artificial Intelligence (AI) methods is that instead of crafting the logic of Artificial Intelligence (AI) using complex behavior trees, Reinforced Learning (RL) rewards the behavior that a person wants the Artificial Intelligence (AI) to manifest, and the agent learns by itself. Then, perform the necessary sequence of actions to achieve the desired behavior. For example, this is how to teach a dog to do tricks using food rewards.
The Reinforced Learning (RL) approach to game Artificial Intelligence (AI) can train a variety of strategic behaviors such as pathfinding, NPC attacking, and defense, and almost any behavior a human can exhibit while playing a video game. State–of–the–art applications include those used to beat best–in–class human players in chess, Go, and multiplayer strategy video games. There are few limits to the strategic behaviors a Reinforced Learning (RL) algorithm can theoretically explore. Still, in practice, the computational cost and environment complexity constrain the types of behaviors it will want to implement using Reinforced Learning (RL).
Reinforced Learning (RL) relies on an agent’s ability to relate things happening in their environment. However, unlike other forms of learning such as classical conditioning, Reinforced Learning (RL) takes it a step further. Behavior is based on the quality and strength of those associations to achieve something desired. Reward (or avoid punishment). In other words, it includes a strategic goal that is optimized over time.
First, there has to be an environment – this is the environment in which learning develops. Then, we can take this one step further into the so–called “global environmental state,” which includes all possible information that can be known about a given environment and the representative’s environmental state, which is a subset of the global climate.
Both open and closed and hidden from players, on the table for players to see. Every location of every card is located in the global environment. Meanwhile, the representative’s climate is characterized by the cards that are revealed to them through their senses, that is, the cards that appear on the table and the cards in their hands. We see only a tiny part of the global ecological situation represented by planet Earth and the universe beyond. If not all, of the information contained in the worldwide environment, and “Markovian” because it provides the ability for the actor to have all the information necessary to be successful in learning the desired target behavior. poker against an alien living on the planet Tao without seeing your cards or knowing whether you’ve won or lost, it would be a hopeless task and utterly “Markovian.”
Another subset of the global environment often used in Reinforced Learning (RL) is the background necessary to make an optimal decision about the future concerning a given goal. In addition, the Markov environmental state summarizes all previous forms of the environment so that no further information is needed to optimize decision–making.